Business analytics has become the backbone of modern organizational decision-making,
transforming raw data into actionable insights that drive success. The ability to analyze complex
datasets, identify patterns, and predict future trends now separates industry leaders from their
competitors.
Key Statistics:
- The global big data market is projected to reach $103 billion by 2027
- Organizations using advanced analytics report 60% higher profit margins
- 94% of businesses say data analytics is crucial to their growth strategy
Business analytics empowers companies to:
- Make data-driven decisions with confidence
- Identify new market opportunities
- Optimize operational efficiency
- Enhance customer experiences
- Reduce business risks
The landscape of business analytics is rapidly evolving. Traditional methods are giving way to
sophisticated technologies like artificial intelligence, machine learning, and real-time analytics.
These advancements are reshaping how organizations collect, process, and utilize data.
Your organization’s ability to adapt to these changes will determine its future success.
Companies that embrace emerging analytics trends and technologies position themselves at the
forefront of innovation. Those that resist change risk falling behind in an increasingly datadriven business environment.
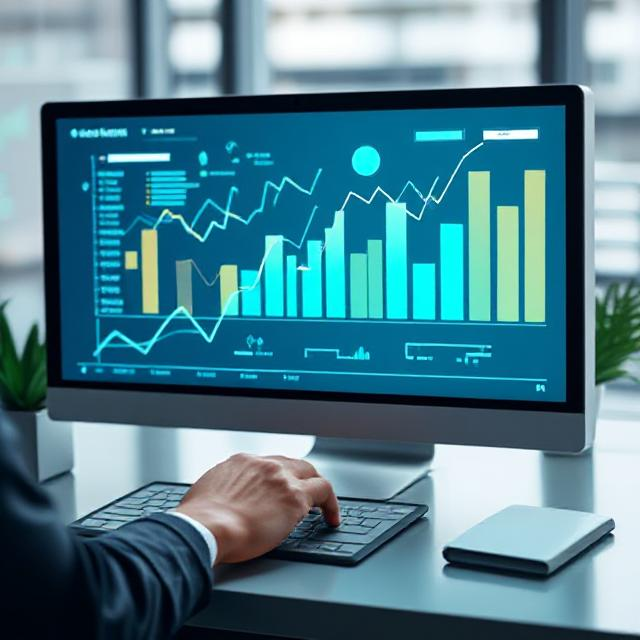
The next decade will bring unprecedented changes to business analytics. Understanding and
implementing these emerging trends isn’t just beneficial—it’s essential for survival in the
modern business landscape.
- How Agentic AI and Emerging Technologies are Changing
Business Analytics
Agentic AI is a game-changer for business analytics. It brings in autonomous systems that can
make decisions and solve problems on their own. Here’s what these AI agents can do:
- Find patterns in complicated datasets without needing human help
- Adjust analytical models instantly based on new data
- Run complex queries using natural language processing
- Provide automated insights and suggestions
When combined with quantum computing, these abilities become even more powerful. Quantum
computing can process huge amounts of data incredibly fast, allowing business analysts to tackle
problems that were once thought impossible:
- Complex Financial Modeling: Running thousands of market scenarios at the same time
- Supply Chain Optimization: Figuring out the best routes among millions of options
- Risk Assessment: Taking into account multiple risk factors in real-time
- Customer Behavior Analysis: Looking at patterns across different demographic groups
AR/VR technologies are also playing a significant role in transforming business analytics. They
create immersive environments for data visualization, changing how businesses interact with
their information:
- 3D visualization of multidimensional datasets
- Gesture-based manipulation of data
- Real-time collaboration in virtual spaces
- Spatial analysis of geographic data
- Virtual boardrooms with live data feeds
- Interactive scenario planning
- Immersive training environments
- Remote team collaboration
These technologies work together to provide powerful analytical capabilities. Here are some
examples:
- A retail business uses agentic AI to understand customer behavior, quantum computing to
optimize inventory scenarios, and AR/VR to visualize store layouts based on foot traffic
patterns. - Manufacturing companies use these tools to simulate production lines, predict when
equipment needs maintenance, and train staff in virtual settings.
The combination of agentic AI, quantum computing, and AR/VR technologies is creating a new
way of looking at business analytics. Organizations can now gain deeper understanding, make
quicker decisions, and see complex data relationships in intuitive ways.
- Ensuring Data Privacy and AI Governance in the Age of Advanced
Analytics
The rapid advancement of AI-driven analytics brings critical challenges in data privacy and
governance. Organizations must implement robust frameworks to protect sensitive information
while maintaining analytical capabilities.
- Regular AI system audits to verify compliance with ethical guidelines
- Clear documentation of AI decision-making processes
- Establishment of AI ethics committees
- Implementation of data handling protocols
- Regular staff training on AI governance principles
Data governance frameworks need to address multiple layers of protection. A comprehensive
approach includes:
- Data Classification SystemsIdentifying sensitive information
- Establishing access levels
- Creating data handling procedures
- Privacy-Preserving TechniquesData encryption at rest and in transit
- Anonymization of personal information
- Secure data storage and transmission protocols
AI models must deliver fair and accurate results across all demographic groups. Organizations
can achieve this through:
- Diverse training data sets representing all user groups
- Regular testing for bias in model outputs
- Implementation of fairness metrics
- Continuous monitoring of model performance
Organizations need transparent processes to build stakeholder trust:
- Clear communication about AI system capabilities
- Regular reporting on AI system performance
- Accessible documentation of data usage
- Open feedback channels for stakeholders
The implementation of blockchain technology adds an extra layer of security and transparency
to data governance. This creates immutable records of data access and modifications, ensuring
accountability in AI-driven analytics processes.
Recent regulations like GDPR and CCPA have raised the stakes for data privacy. Organizations
must adapt their governance frameworks to meet these evolving requirements while maintaining
analytical capabilities. This includes implementing privacy-by-design principles and conducting
regular privacy impact assessments.
Democratizing Access to Insights with No-Code Solutions and Self-Service Analytics
The rise of no-code and self-service analytics platforms has transformed the data analytics
landscape, breaking down traditional barriers between technical experts and business users.
These intuitive tools enable professionals across departments to harness data insights without
extensive programming knowledge.
- Drag-and-drop interface builders
- Visual data modeling platforms
- Automated report generators
- Pre-built analytics templates
- Interactive dashboard creators
Business users can now perform complex data analysis tasks through user-friendly interfaces:
- Data exploration and visualization
- Custom report creation
- Real-time dashboard monitoring
- Basic predictive modeling
- Automated insights generation
- Faster Decision Making
- Reduced dependency on IT departments
- Immediate access to insights
- Quick response to market changes
- Cost Efficiency
- Lower training requirements
- Reduced need for specialized staff
- Decreased technical debt
- Enhanced Collaboration
- Shared dashboards and reports
- Cross-departmental data access
- Unified data interpretation
- Tableau
- Power BI
- Looker
- Sisense
- Qlik Sense
These platforms incorporate AI-powered features to suggest relevant visualizations and
highlight key patterns in data. Users can create sophisticated analyses through intuitive
interfaces while maintaining data governance standards.
The democratization of analytics has sparked a cultural shift in organizations. Teams now
approach decision-making with data-driven mindsets, leading to improved operational efficiency
and innovation across all business functions.

Modern self-service analytics platforms also feature built-in data quality checks and automated
data preparation tools, ensuring accuracy while reducing the technical expertise required for
meaningful analysis.
- Proactive Decision-Making through Predictive and Prescriptive
Analytics Techniques
Predictive and prescriptive analytics represent a significant shift from reactive to proactive
decision-making strategies. These advanced techniques enable businesses to anticipate future
outcomes and optimize their operations before issues arise.
- Supply Chain Optimization: Major retailers like Walmart use predictive models to
forecast inventory needs, reducing stockouts by 16% - Equipment Maintenance: Manufacturing companies implement predictive maintenance,
cutting downtime by up to 50% - Customer Behavior: Banks leverage predictive analytics to identify potential churners
with 85% accuracy - Route Optimization: UPS saves millions annually using prescriptive analytics to
determine optimal delivery routes - Price Optimization: Airlines adjust ticket prices in real-time based on demand patterns
- Resource Allocation: Healthcare facilities optimize staff scheduling during peak times
- Risk assessment through multiple “what-if” scenarios
- Strategic planning with data-backed confidence
- Resource optimization across different potential outcomes
Netflix saves $1 billion annually through predictive analytics in content recommendations
Amazon’s prescriptive analytics reduce shipping costs by 10-40% while improving delivery times
- Advanced machine learning algorithms
- Real-time data processing capabilities
- Integration with existing business systems
- Automated decision-making protocols
These techniques transform raw data into actionable insights, enabling businesses to make
informed decisions before market changes occur. Companies implementing predictive and
prescriptive analytics report a 20-30% improvement in operational efficiency and a 15-25%
increase in revenue growth.
Instant Insights with Real-Time Analytics Powered by Edge Computing
Real-time analytics has transformed how businesses make decisions by allowing them to process
and analyze data as it is created. With edge computing infrastructure, the power to compute is
brought closer to where the data is generated, minimizing delays and enabling quick responses
to changing situations.
- Immediate detection of anomalies and threats
- Dynamic pricing adjustments based on market conditions
- Instant customer experience personalization
- Rapid response to equipment maintenance needs
- Real-time inventory management
A leading automotive manufacturer implemented edge computing-powered real-time analytics
across its production facilities. The system processes data from thousands of IoT sensors,
enabling:
- 35% reduction in equipment downtime
- 28% improvement in production efficiency
- Real-time quality control adjustments
- Predictive maintenance scheduling
A major retail chain deployed real-time analytics for its in-store operations and e-commerce
platform: - Dynamic pricing updates based on competitor actions
- Instant inventory level adjustments
- Real-time personalized recommendations
- 42% increase in customer engagement
The combination of 5G networks and edge computing has enhanced the capabilities of real-time
analytics even further. Organizations can now process large volumes of data right at the source,
reducing the need for extensive bandwidth and enabling quicker decision-making.
When implementing real-time analytics powered by edge computing, organizations should keep
the following factors in mind:
- Data quality monitoring
- Edge device management
- Network reliability
- Security protocols
- Scalability planning
Real-time analytics powered by edge computing has transformed industries from healthcare to
finance. Healthcare providers use it for patient monitoring and treatment optimization, while
financial institutions leverage it for fraud detection and trading decisions. The technology
continues to evolve, offering new possibilities for businesses to gain competitive advantages
through instant data-driven insights.
Additional Emerging Trends Shaping the Future Landscape of Business Analytics
The business analytics landscape continues to evolve with innovative trends reshaping how
organizations leverage data for competitive advantage. Here are the key developments driving
this transformation:
- AI-powered algorithms analyze individual customer behaviors, preferences, and
interactions - Real-time customization of product recommendations and service delivery
- Dynamic pricing strategies based on customer segments and market conditions
- Personalized content creation and distribution across multiple channels
- Carbon footprint tracking and reduction strategies
- Supply chain optimization for environmental impact
- ESG (Environmental, Social, and Governance) performance metrics
- Resource consumption analysis and waste reduction planning
- Automated resource scaling based on usage patterns
- Intelligent workload distribution across cloud provider
- Cost prediction models for budget planning
- Waste identification and elimination in cloud services
- Advanced algorithms combining multiple data sources
- Automated decision-making for routine operations
- Risk assessment and mitigation strategies
- Integration with existing business processes
- Unified data management across distributed environments
- Seamless data integration from multiple sources
- Automated data discovery and cataloging
- Enhanced data accessibility and security
These trends are driving organizations to adopt more sophisticated analytics capabilities.
Companies implementing these technologies report:
- 25% increase in customer engagement through hyper-personalization
- 30% reduction in cloud computing costs
- 40% improvement in sustainability metrics
- 35% faster decision-making processes
The adoption of these emerging trends requires organizations to invest in new tools and
technologies while developing specialized expertise in these areas. Companies that successfully
integrate these capabilities gain significant advantages in market responsiveness and operational
efficiency.
The Integration Challenge: Balancing Human Expertise with AIEquipped BI Tools
The combination of human expertise and AI-powered Business Intelligence tools creates a
powerful partnership, but also presents unique challenges that organizations must overcome.
This integration turns traditional reporting systems into dynamic analytical powerhouses.
- Automated Pattern Recognition: AI algorithms detect subtle data patterns humans
might miss - Enhanced Decision Speed: Real-time insights enable faster strategic choices
- Reduced Manual Work: Automation of routine analytical tasks
- Scalable Analysis: Processing of massive datasets without human limitations
- Training requirements for existing staff
- Need for specialized AI-BI hybrid roles
- Resistance to new technological adoption
- Balancing AI recommendations with human judgment
- Establishing validation protocols for AI outputs
- Creating accountability frameworks
- Phased Implementation
- Start with pilot projects
- Gradually expand AI capabilities
- Build user confidence through small wins
- Hybrid Decision-Making Model
- AI handles data processing and initial analysis
- Human experts provide context and final decisions
- Regular review of AI-human collaboration effectiveness
- Continuous Learning System
- Regular updates to AI models based on human feedback
- Documentation of successful integration cases
- Adaptation of workflows based on user experiences
The key lies in creating a balanced ecosystem where AI augments human capabilities rather than
replacing them. Organizations successfully navigating this integration often maintain clear roles
for both AI systems and human experts, ensuring each contributes their unique strengths to the
analytical process.
Using Speech Analytics to Improve Customer Interactions Management
Speech analytics technology has changed the way customer interactions are analyzed by turning
raw conversation data into useful business intelligence. This powerful tool captures and analyzes
customer conversations across multiple channels, including phone calls, video meetings, and
voice messages.
- Real-time sentiment detection identifies customer emotions and satisfaction levels
- Pattern recognition reveals common issues and emerging trends
- Automated quality monitoring ensures compliance and service standards
- Sales opportunity identification through keyword tracking
- Risk mitigation by flagging potential customer churn signals
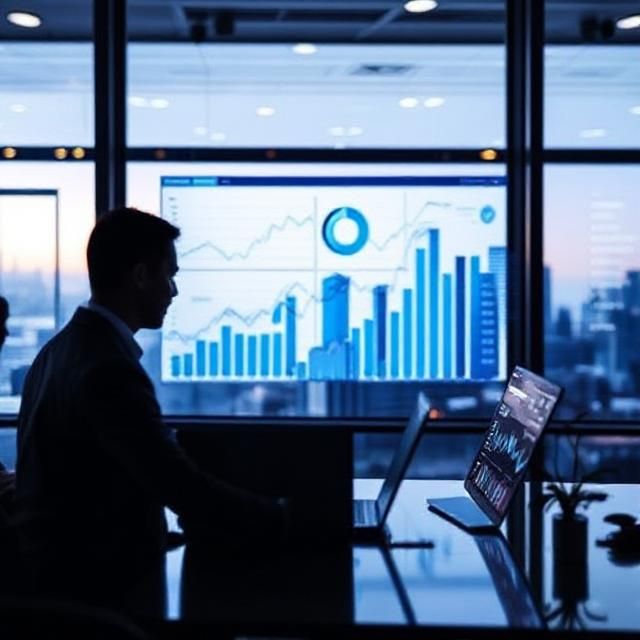
The implementation of speech analytics drives revenue growth through targeted strategies based
on actual customer feedback. Companies using speech analytics report a 15-20% increase in
customer satisfaction scores and a 10-25% reduction in average handling time.
- Emotion Detection: AI algorithms analyze voice patterns, tone, and pitch to gauge
customer emotions - Intent Recognition: Identifies customer needs and potential sales opportunities
- Topic Categorization: Automatically groups conversations by common themes
- Compliance Monitoring: Ensures adherence to regulatory requirements
- Performance Metrics: Tracks agent effectiveness and customer engagement
Speech analytics also enables personalized customer experiences by providing insights into
individual preferences and behavior patterns. Organizations can create targeted marketing
campaigns and customize service delivery based on analyzed conversation data.
- Call centers use speech analytics to identify training needs and improve agent performance
- Sales teams leverage conversation insights to refine pitching strategies
- Product development teams gather customer feedback for feature improvements
- Marketing departments analyze customer language patterns for campaign optimization
The technology’s accuracy continues to improve with advancements in natural language
processing and machine learning algorithms. Modern systems can achieve up to 95% accuracy
in transcription and analysis, even with challenging accents or background noise.
Overcoming Data Security Risks and governance Challenges in an Evolving landscape
Data security is crucial in today’s business analytics world. Companies need to have strong
security measures in place to protect their valuable data while also following constantly
changing regulations.
- Data Encryption – Implementing end-to-end encryption for data at rest and in transit
- Access Control – Establishing role-based access management systems
- Regular Audits – Conducting periodic security assessments and vulnerability tests
- Incident Response Plans – Creating detailed protocols for potential data breaches
The introduction of GDPR and similar regulations has changed the way organizations handle
data governance. They now require strong frameworks that cover:
- Data Collection Transparency
- User Consent Management
- Right to Data Erasure
- Data Portability
- Privacy Impact Assessments
- AI-powered cyber threats
- Cloud storage vulnerabilities
- Third-party vendor risks
- Remote workforce security concerns
Organizations can improve their security measures by using a “Security by Design” approach,
which means including security measures in analytics processes from the beginning instead of
adding them later.
- Deploy automated security monitoring tools
- Establish clear data classification guidelines
- Create comprehensive audit trails
- Implement regular staff training programs
- Develop incident response procedures
The rise of edge computing and distributed analytics has brought about new security challenges.
Organizations need to adjust their security protocols to protect data across multiple locations
while still being efficient in processing.
- Regular security updates and patches
- Multi-factor authentication
- Zero-trust security models
- Continuous monitoring systems
- Data backup and recovery plans
These security measures must constantly evolve to keep up with the rapid technological
advancements in business analytics. Companies need to find a balance between strong security
protocols and operational efficiency in order to stay competitive.
Edge Computing: Boosting Operational Efficiency
Edge computing is changing the game for businesses by processing data closer to where it
comes from. This means faster decision-making and smoother operations across various
industries.
In simple terms, edge computing brings the power of computing right to the source of data.
Instead of sending all data to a central server for processing (which can be slow and inefficient),
edge computing allows devices or local servers to handle some of that processing. This has
several advantages:
- Speed: By reducing the distance data has to travel, response times can be significantly
improved. - Bandwidth Savings: Less data being sent over the network means lower bandwidth costs.
- Reliability: In cases where internet connectivity is unreliable, local processing ensures
that operations can continue even without a connection.
The benefits of edge computing are not just theoretical. Many businesses have already seen
significant improvements in their operations after adopting this technology:
- A manufacturing plant using an edge-enabled quality control system was able to detect
defects with 95% accuracy, leading to higher product quality and fewer returns. - A retail chain implemented edge computing for inventory management and saw a 30%
increase in accuracy, resulting in better stock availability and reduced wastage.
These examples highlight how edge computing can directly impact key performance indicators
(KPIs) such as defect rates or inventory accuracy.
While edge computing has potential applications across various sectors, certain areas are
particularly well-suited for its implementation:
- Manufacturing: Real-time monitoring of equipment performance and predictive
maintenance using sensors connected via edge devices. - Retail: Instantaneous tracking of inventory levels through RFID tags read at the point-ofsale or distribution centers.
- Healthcare: Continuous monitoring of patients’ vital signs using wearable devices with
local processing capabilities. - Finance: High-frequency trading algorithms running on nearby servers to minimize
latency compared to traditional cloud solutions. - Logistics: Optimizing delivery routes based on traffic conditions analyzed locally by GPS
devices.
These use cases demonstrate how different industries can leverage edge computing to address
specific challenges they face.
As more organizations recognize the value of operational efficiency improvement, we can
expect further adoption of technologies like edge computing. By bringing computation closer to
where it matters most – whether that’s a factory floor or a retail store – businesses stand to gain
significant advantages over their competitors.
Continuous Learning: A Key Imperative for Business Analytics Professionals
The rapid evolution of business analytics requires professionals to adopt a mindset of continuous
growth. Your success in this field depends on staying up-to-date with new technologies,
methods, and industry best practices.
- Advanced data visualization techniques
- Machine learning algorithms and AI applications
- Cloud-based analytics platforms
- Programming languages (Python, R, SQL)
- Statistical analysis and modeling
- Data governance and security protocols
Organizations understand the importance of upskilling employees through structured learning
programs. Companies such as Google, Amazon, and Microsoft offer specialized certifications
designed for business analytics professionals. These programs offer practical experience with
state-of-the-art tools and real-world applications.
- Attending industry conferences and webinars
- Becoming a member of professional networks and communities
- Participating in peer-to-peer learning sessions
- Enrolling in online courses from platforms like Coursera and edX
- Working on hands-on projects to implement new skills
The investment in continuous learning brings about concrete advantages:
- Enhanced problem-solving abilities
- Improved accuracy in decision-making
- Increased opportunities for career advancement
- Better adaptability to technological changes
- Stronger competitive edge in the job market
Professional development in business analytics requires a balanced approach that combines
technical expertise with business understanding. Knowing both areas allows you to provide
valuable insights that contribute to the success of the organization.
Conclusion
The world of business analytics is constantly changing, and organizations and professionals need
to be proactive in order to keep up. Success depends on being able to use new technologies
while also having strong data management practices in place.
Here are the key factors that will determine success in today’s business analytics:
- Quick Adoption of Technology – Organizations need to quickly adopt tools like AI, edge
computing, and advanced visualization techniques. - Data-Driven Culture – Companies should create an environment where data literacy and
analytical thinking are essential skills. - Focus on Security – It’s important to have strict data protection measures in place while
still allowing access to information. - Balanced Integration – Decision-making should involve both human expertise and AI
capabilities.
The future will belong to organizations that can combine technological innovation with strategic
insight. Those who are able to find this balance will discover new opportunities, drive growth,
and stay ahead of the competition in a business world that is increasingly driven by data.
Your analytics strategy needs to continuously evolve – staying the same means falling behind.
Are you ready to embrace the future of business analytics?